Understanding AI and How It Sources Information
The Complexity of AI Information Sourcing: Risks, Challenges, and Best Practices for Marketers
Artificial Intelligence (AI) has transformed how we access and process information. From search engines to chatbots, AI-driven platforms synthesise vast amounts of data to provide seemingly instant answers. However, the way AI sources and verifies information is far from straightforward. Different platforms rely on varied methodologies, ranging from pre-trained models and proprietary databases to live web scraping and real-time data indexing.
The Marketing Made Clear Podcast
This article features content from the Marketing Made Clear Podcast – check it out on all good platforms.
How ChatGPT Sources Information vs. Other AI Platforms
ChatGPT (including its iterations like GPT-4) is based on a large language model (LLM) trained on a mixture of publicly available text, licensed data, and OpenAI’s proprietary datasets. However, it does not access live internet data in real-time unless integrated with a web browsing tool. Instead, responses are generated based on pre-existing training data and pattern recognition. This means that while ChatGPT can provide extensive insights based on its training, it cannot verify or update information dynamically without external input.
Google Search and Generative AI Models: AI-powered search engines, such as Google’s Search Generative Experience (SGE), rely on indexing billions of web pages, ranking them based on relevance and authority. However, AI-generated responses in search results may sometimes present summarised insights from unreliable sources, leading to information distortion.
Bing AI & Other Internet-Connected Chatbots: Some AI models, like Microsoft’s Bing AI, have access to real-time web searches, allowing them to pull the latest information. However, even with this capability, the risk of misinformation persists if these tools prioritise popularity over accuracy.
The Risk of AI Creating a Self-Perpetuating Rhetoric
A critical issue with AI-generated content is the potential for self-perpetuating misinformation cycles. This occurs when incorrect or misleading AI-generated responses are published online, later to be re-indexed and used as sources by other AI models. This feedback loop can lead to the reinforcement of false narratives, making it increasingly difficult to distinguish between factual and fabricated information.
Case Study: AI and the Mandela Effect in Digital Information
The Mandela Effect, where large groups of people misremember facts, is an apt metaphor for AI’s challenges with misinformation. If an AI model incorrectly claims a historical event happened in a specific way, and that information is then cited by another AI model, over time, the false claim could gain credibility purely due to its repeated digital presence.
This risk is heightened in marketing, journalism, and academia, where AI-generated insights may shape decision-making processes based on incorrect data.
The Role of Peer-Reviewed Academic Works in Addressing AI Misinformation
One potential solution to AI misinformation is the reliance on peer-reviewed academic literature. Peer-reviewed research follows stringent verification processes, including scrutiny by subject-matter experts, rigorous testing, and replication of findings. If AI platforms were designed to prioritise sourcing information from such verified academic databases (e.g., Google Scholar, PubMed, or ResearchGate), the risk of misinformation could be significantly reduced.
However, integrating AI with academic verification is challenging due to:
-
Access Restrictions: Many peer-reviewed journals are behind paywalls, limiting AI’s ability to reference them.
-
Complexity of Academic Language: AI must interpret dense academic papers and translate findings into digestible insights without oversimplifying or misrepresenting the data.
-
Time Lag in Research Publication: Academic papers often take years to publish, whereas AI-driven content demands real-time updates.
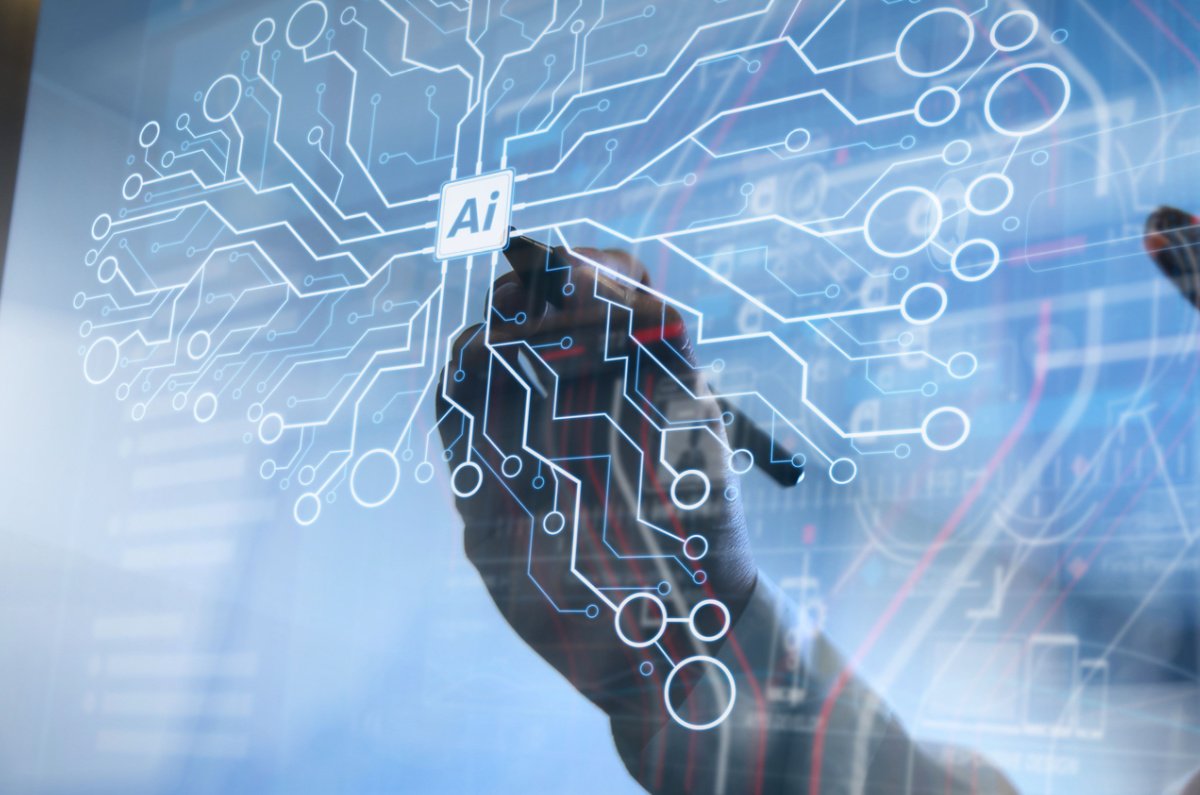
What Marketers Can Do to Use AI Responsibly
Marketers are increasingly using AI for content creation, audience insights, and trend analysis. However, they must be aware of AI’s limitations and take active steps to ensure they are not inadvertently perpetuating misinformation. Here are key best practices:
-
Cross-Check AI Outputs with Verified Sources: Always validate AI-generated insights against reputable sources, such as government reports, academic publications, and industry-leading research.
-
Utilise Authoritative Databases: When possible, leverage trusted databases like Google Scholar (although even this is debatable), FactCheck.org, or academic journals rather than relying solely on AI outputs.
-
Monitor AI-Generated Content for Bias and Errors: AI models can inadvertently produce biased or misleading content. Reviewing outputs critically is crucial before publishing or sharing AI-driven insights.
-
Stay Updated on AI Developments: AI platforms continuously evolve, and understanding how they source information can help marketers use them more effectively.
-
Encourage Transparency in AI-Sourced Content: If using AI-generated content in marketing materials, disclose the source and methodology behind the insights.
Conclusion
AI is a powerful tool, but it is only as reliable as the data it is trained on. Without proper verification mechanisms, the risk of misinformation grows, potentially leading to a self-reinforcing loop of false narratives. Marketers must take an active role in validating AI-generated content, prioritising peer-reviewed academic research and reputable sources to maintain credibility and accuracy in their work. By using AI responsibly, marketers can harness its potential without falling into the trap of misinformation.